Jon: Why I joined Bigeye
Jon Hsieh, product manager at Bigeye, talks about why he joined the company and what excites him about the opportunity.
.webp)
Get the Best of Data Leadership
Stay Informed
Get Data Insights Delivered
About a month ago, I had the good fortune to start my next career adventure on the product team at Bigeye and help build the next generation of data engineering tools. Here’s why I chose to join Bigeye and why this opportunity has me really excited. It boils down to three main reasons: 1) the need for data reliability, 2) the vision of the company’s leaders, and 3) the company culture.
The need for a data reliability platform
I joined Bigeye in part to work on one of the biggest challenges and opportunities facing data today: data reliability. Data observability is the ability to have constant and complete knowledge of what’s happening inside all the tables and pipelines in your data stack—a need that is both mission-critical and inevitable.
Let’s start with Monica Rogati’s Data Science hierarchy of needs, one of my favorite diagrams, which illustrates the needs of a modern data organization. It explains that if a business wants to use AI and deep learning, they need to build a reliable base using the tech from the layers below it. With this in mind, how far are we today?
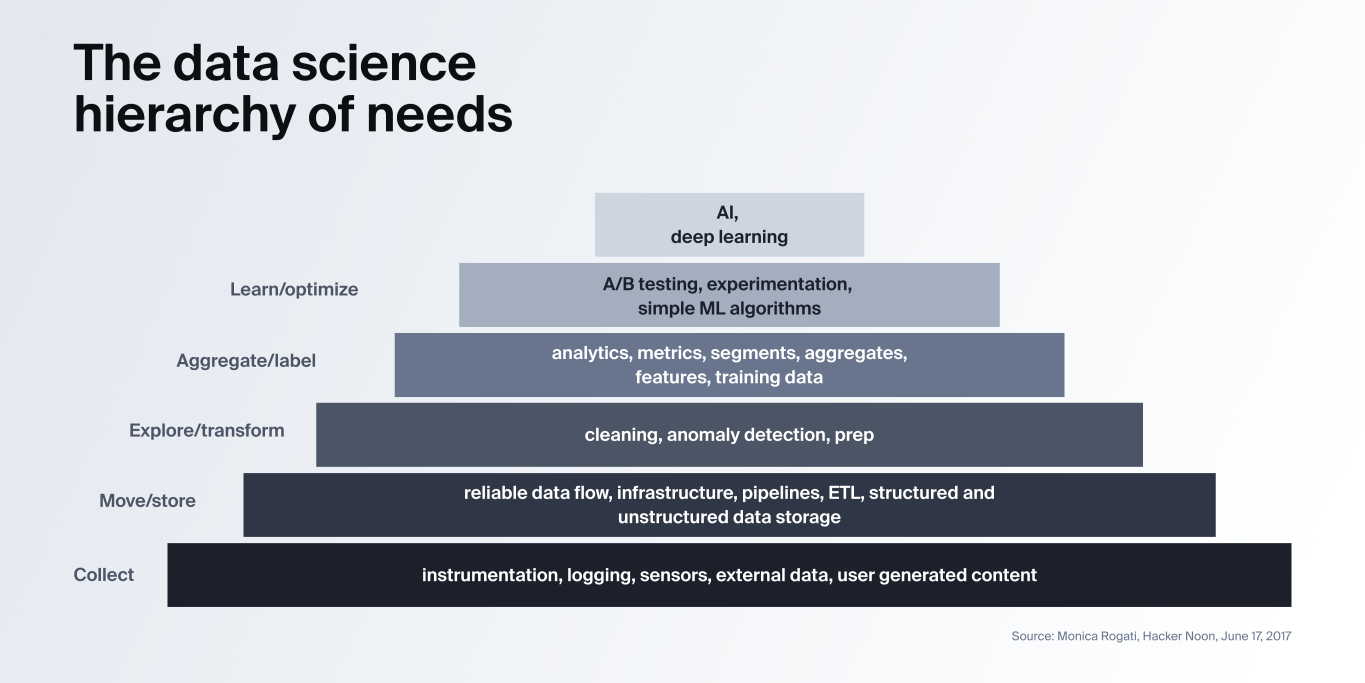
Over the past decade, while at Cloudera, I focused on the bottom two layers, building out big data infrastructure. Most of the light and heat have been around big data systems (Hadoop, Spark, Snowflake, Kafka) that enable reliable movement, storage, and collection of data. Today these two bottom layers are well-established and a small team, at almost any company, could spin up a scalable data warehouse or data lake in the cloud in a few days.
When you consider the top of the pyramid, there have been many ML/AI companies and efforts, but many are niche products that solve specific problems that aren’t applicable to a majority of enterprises. Unfortunately, it seems that many companies who want to use AI and ML on their data can’t seem to reach that level. A layer or two down on the analytics front, the data mesh concept has recently become popularized. Even that is hard to achieve. Why is this?
The bet that I’m making is that it is because of the middle layer.
The “explore/transform” layer which includes cleaning, anomaly detection, and prep is critical, but this layer hasn’t had the emphasis, hype, or funding to mature as quickly as the other layers have. Because the bottom layers have become easy enough for most teams to implement and deploy, the timing is right for solving the middle layer to fully unlock all the layers to all enterprises.
Fundamentally, the disconnect between the top layers and bottom layers is the ability to rely upon the data. Ten years ago, Gartner stated that 40% of companies can’t even get to reliable analytics. For most organizations today, I don’t think we are much better off. And that’s why the focus on the systems needs to shift to a focus on the data and the people using it.
There are many companies that rely on data to run and grow their businesses, and the successful ones have built their own tools for ensuring data reliability. In these cases, each has built out a bespoke system and can be resource-intensive to maintain even within their own organization. A better approach is to instead have a product or platform that standardizes and automates the way data reliability problems get solved. In fact, it’s a prerequisite that enables data analysts to trust the dashboards their execs rely upon, enables data teams that want to move towards a data mesh to share data with other data engineering teams, and enables data scientists working on ML/AI models to have confidence they have clean data.
The vision
As you might imagine, many companies are now trying to tackle data reliability. So why Bigeye?
During my research and the interview process, only a few companies stood out and shared the vision for data and data reliability that I had been contemplating. When I had my first chats with Bigeye’s founders, Kyle and Egor, it became clear that I had found kindred thinkers who also had a strategic mindset and experience to back it up.
While at Cloudera, I maintained a set of data pipelines. Like folks at many other companies, I built a basic data quality and monitoring system for the data pipelines. There were a few key insights from this experience:
- Data engineering is 10-20 years behind software engineering. Key DevOps ideas like CI/CD, testing, and observability are critical for repeatedly developing production services, and the ideas behind these practices are needed for DataOps with production data pipelines and datasets.
- Data monitoring and data quality testing are analogous to software testing and software observability. For example, data sets have schemas and invariants, just like software has API’s with types and invariants.
- It’s a decoupling world where the best of breed wins. Gone are the days when a single company can be best of breed at all levels of the stack. Today’s “modern data stack” is a common diagram with multiple contenders in each discipline.
While Kyle and Egor were at Uber, they had similar insights. They had led and built out more than just data testing and data quality tools; they built out a full data observability platform that could handle orders of magnitude more scale, more people, and more comprehensive tooling than my little bespoke project. While I had seen the possibilities, they had been there before, built it, and knew where the pitfalls were.
It wasn’t just me who saw this. Several top-tier VCs including Sequoia, Coatue, and Costanoa are helping Bigeye turn its vision into reality. These investors have been in the startup and data space for a long time, and they have the resources and connections to build a successful company. If the founders could build the team to execute, the vision is in reach. That’s a ride that I want to be part of.
The culture
So, about the team. For me, a deciding factor is company culture. While the market and the vision may evolve over time, the DNA of a startup comes from the founders, their values, the people they attract, and the culture they create. Clear even from my initial conversations, this was a team that had many cultural elements I was looking for.
The first thing that struck me was how open and candid the team was. These were people on a mission. Instead of being cagey, they were open when they discussed their thesis, the vision, and parts of the strategy. To me, this kind of pitch is very compelling—enough to take a leap of faith from a comfortable job into the startup world.
Bigeye has a strong product and engineering culture, but they also have a broad set of talents and teammates from many backgrounds. Sure, several folks followed the founders from Uber, but the team is made up of folks with experience at all levels of the data stack (Amazon, Snowflake, Alation, Confluent), enterprise operations companies (Lightstep, Sumologic, Servicenow), and organizations where data reliability is critical (NASA, Collective Health). Many of us have seen and led through times of hyper-growth—from Seed to Series A to post-IPO. This diversity of backgrounds and relationships will help the company as we integrate with the other parts of the modern data stack.
One final aspect that came out during the process is how fairness is baked into the values of the culture. When tough decisions need to be made, the facts are laid out, there is thoughtfulness in explaining the "why" behind decisions, and the team sticks to their principles. I deeply respect that and feel that that approach is important to maintain the core of its culture as it scales.
I’m here
So, I’ve done it. I’ve joined Bigeye to tackle the data reliability problem. It is an area ripe for solutions and a nascent market that I’m convinced is about to break out. Solving data reliability is a prerequisite for making analytics trustworthy and AI/ML usable by all enterprises. Just as important, Bigeye is a company with the vision, culture, and team that will enable it to swing for the fences and make magic with data.
Interested in learning more? Feel free to reach out to our CEO and co-founder Kyle Kirwan and request a demo to see Bigeye in action.
Monitoring
Schema change detection
Lineage monitoring